Prediction of Rainfall-INduced landslides – Improving multi-scale TerritoriAL Early warning through aRTificial intelligence
(PRIN-ITALERT)
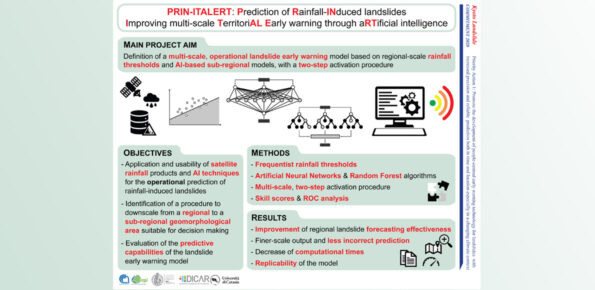
Background
Predicting the occurrence of rainfall-induced landslides is a crucial task for civil protection purposes. Several landslide early warning systems are currently operating worldwide at different spatial scales. In Italy, landslide alerts are issued for territorial units named Alert Zones, covering a few thousand km². Usually, regional-scale warning models rely on rainfall thresholds, which require a few parameters and provide clear results in short computational times. However, a regional-scale model at the alert zone scale may miss some local features that could be relevant for landslide initiation. This could hamper an effective warning by producing incorrect predictions. For this reason, a multi-scale approach is required.
Purpose
The PRIN-ITALERT project (Prediction of Rainfall-INduced landslides – Improving multi-scale TerritoriAL Early warning through aRTificial intelligence) aims at improving the operational prediction of rainfall-induced landslides over large areas taking advantage of both rainfall thresholds defined using satellite-based rainfall products and innovative approaches based on artificial intelligence algorithms, in particular, Random Forest and Artificial Neural Networks.
Methods
Usually, regional-scale LEWS rely on rainfall thresholds, which are mainly based on the statistical analysis of the rainfall conditions responsible for recorded landslides. Satellite-based rainfall products were increasingly employed in the definition of rainfall thresholds and were proved to provide good performance in landslide prediction.
Among the several AI techniques, Artificial Neural Networks (ANN) and Random Forest (RF) are among the most used algorithms in landslide hazard assessment and prediction. ANN were recently applied for the purpose of improving landslide prediction models at local and regional scales, while several applications to landslide susceptibility mapping appeared starting from about two decades ago. RF was proved to be one the best algorithms for landslide susceptibility assessment and it was also used for landslide detection, or prediction of landslide movements from rainfall data.
Results
The main objective of PRIN-ITALERT is the definition of a multi-scale operational landslide prediction model based on regional-scale rainfall thresholds and AI-based sub-regional models, with a 2-step activation procedure. The rainfall thresholds are used as a first operational step of a multi-scale prediction model integrating AI-based algorithms, which will be activated only in case of threshold overcoming. The combination of both tools has the advantage of: 1) increasing the predicting effectiveness at a finer spatial resolution compared to the traditional regional-scale models; and 2) reducing the computational times.
Conclusions
The project is expected to have relevant scientific, operational, and social impacts. A significant advancement of the state of the art concerning the spatial and temporal prediction of rainfall-induced landslides is foreseen; an improvement in the predictive capabilities of regional-scale models, also thanks to the multi-scale approach is expected, with a finer-scale output and a reduced lead-time, useful in hazard management and early warning activities. A key aspect of the proposal is that the developed methodology can be applied to other landslide-prone areas of the globe, as remote sensing rainfall data are now available in nearly real-time on a grid of global coverage.